Generative AI in Banking: Transformative Potential and Game-Changing Use Cases
Generative AI isn’t just a buzzword – It is a powerful game-changer transforming the banking industry. Real-life use cases have shown how Gen AI is reshaping the industry.
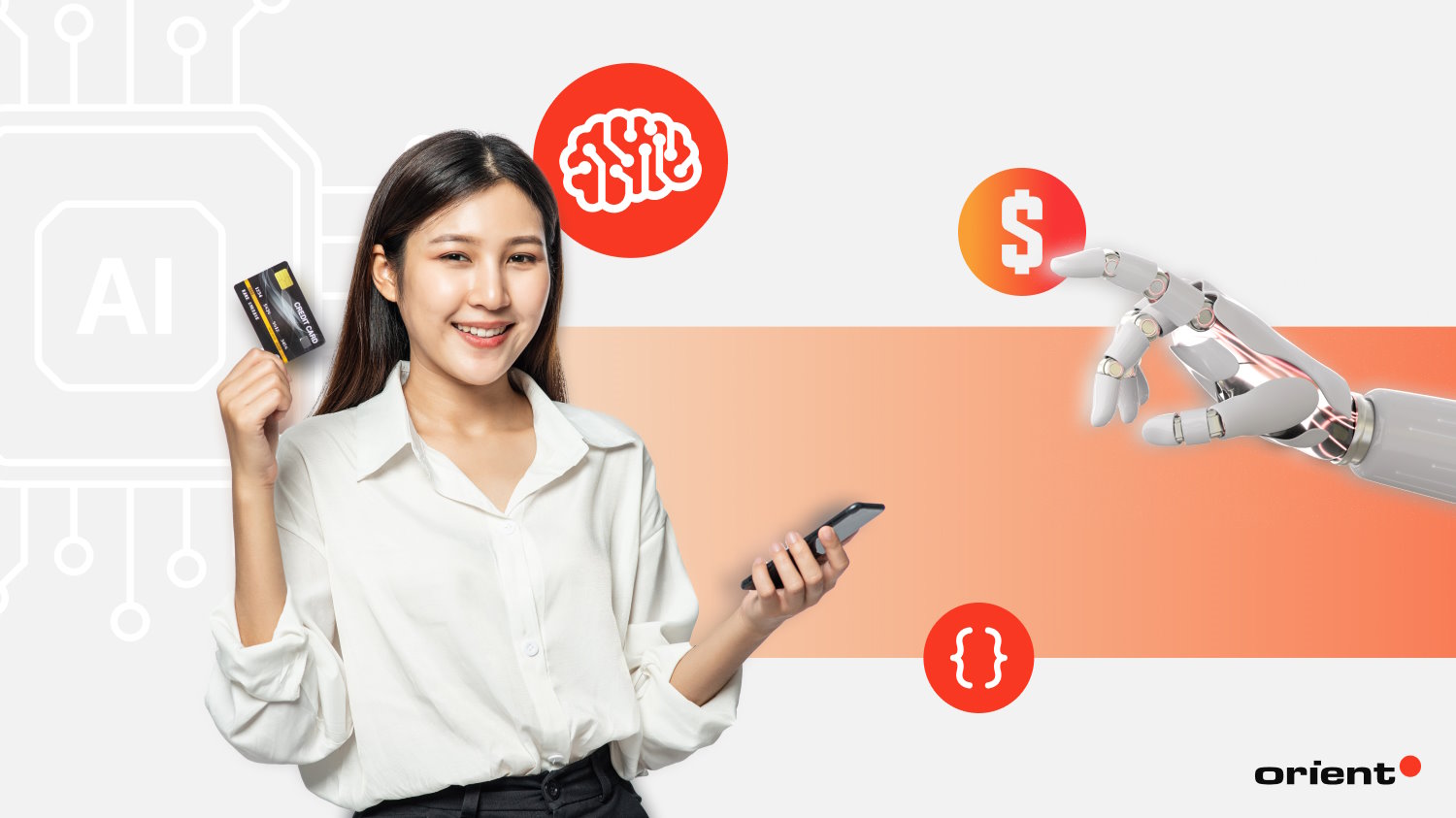
Content Map
More chaptersGenerative Artificial Intelligence isn’t just a buzzword – It’s a powerful game-changer transforming the banking industry. Real-life use cases are showing how Gen AI is reshaping the industry.
Generative AI is only starting to make its way into the mainstream world, but it has already made a significant impact on every industry, and banking is certainly no exception. It’s slowly becoming a necessity rather than a “maybe future technology.”
To stay competitive in today’s complex technological landscape, banks need to adapt and evolve constantly. After all, modern technology is the core that drives growth transformation. What’s even more important, however, is understanding how generative AI can support or optimize a business and its operation.
This article aims to shed light on how generative AI is transforming the banking sector, why it matters at all, and its potential use cases and limitations.
Key Takeaways:
- AI has been part of the banking industry for years, but the emergence of generative AI has asked banks to embrace the new technology to stay competitive.
- The push for the adoption of generative AI isn’t to only stay competitive but also to enhance the overall operation and customer experience.
- Generative AI in banking use cases can be categorized into four main types, including customer engagement, risk management and operation, strategic decision-making, and process optimization.
- While some banks are already developing and using the tech in their day-to-day operations, organizations need to think of the most suitable operational generative AI archetype, from being centralized to completely decentralized.
AI Evolution in the Banking Section
Banks are no strangers to artificial intelligence (AI) and machine learning (ML). Their fluid business model, the massive amount of data, and repetitive data processing tasks are partly why they are such eager early adopters.
Banks are known for using traditional AI to perform tasks involving data classification, process automation, and detection of hidden patterns. Traditional AI, or Narrow AI, refers to systems designed to perform specific tasks intelligently by learning from data and making decisions or predictions within predefined rules. In the late 2000s, banks utilized ML models, both supervised and unsupervised, to perform banking tasks, e.g., using the Python programming language for stronger data analytics or machine learning libraries like SKLearn and TensorFlow for faster data structuring and analysis.
It is only natural that banks are ready to adopt the next AI generation: Generative AI. However, as Gen AI is only in its infancy, the tech still needs further testing and experimenting. One thing is for sure, though, that the emergence of gen AI is expanding and revolutionizing the use of AI in banking.
Why Generative AI in Banking Matters
Facing the strong rising tidal waves of generative AI, it’s easy for banking organizations to jump right on the trend. It is also understandable, however, if you are hesitating and need clarification on this technology. The following are several reasons that prove the importance of this technology in the banking industry.
- The wave of new-generation AI technology brings major opportunities to financial technology (fintech) organizations. Even though fintech has been steadily developing over the recent years, AI will magnify fintech’s ability to disrupt the industry. As a result, banks are pressured to test and explore Gen AI’s capabilities.
- Gen AI can produce natural, human-like responses, which is why it’s often used in chatbots or virtual assistants. This ensures 24/7 enhanced customer service and satisfaction.
- AI transforms financial institutions’ operations by automating tasks like data entry and document processing with high accuracy.
- Paired with other technologies like cloud computing or big data, AI uncovers hidden patterns and trends, allowing banks to make informed and confident decisions. Analyzing a large amount of data helps banks detect suspicious patterns and take prompt action.
- By analyzing the rich data, AI can identify the gap in banking products and suggest improvements or new products to meet current customer demands and stay competitive.
Gen AI in Banking: Practical Use Cases
Even though it seems like AI is rapidly taking over every single facet of banking, Gen AI is only in its early development stages. Still, it helps to examine AI key use cases, so you have a better idea of how this technology is applied in real life.
Customer Engagement
Gen AI is steadily reshaping how banks interact with customers. Every email drafted, and every notification sent will be more personalized and targeted. Relationship managers will have a powerful tool to draft meaningful outreach messages in a matter of seconds. Customers no longer have to wait until working hours to receive personalized financial advice.
Customer Service and Support
Gone are the days of visiting brick-and-mortar stores to view products or services. Technology has made 24/7 access to a business possible. Web pages and mobile apps strengthen businesses’ connection with customers.
Still, 24/7 access doesn’t always equal 24/7 customer support. This is where AI chatbots come in and disrupt the industry. Thanks to large language models (LLM) that understand user’s intent, your customers can have natural-sounding conversations with chatbots or virtual assistants via email or chat. An AI-powered chatbot can introduce and recommend financial products, check account balances, or initiate transactions.
As a matter of fact, several banks already have chatbots in place. For example, Ally Assist from Ally Bank is one of the first AI banking chatbots. Bank America’s chatbot, Erica, serves as a personal assistant, providing helpful personal insights. Eva by HDFC is known for its sophistication and high-accuracy answers. Chatbots aren’t a new technology in banking, but Generative AI has elevated them to a new level of complexity and natural-sounding answers.
Personalized Marketing
Utilizing gen AI technology, banks analyze customer data speedily to gain valuable insights into customer personas, preferences or changing demands. This information allows banks to personalize their marketing efforts, e.g. personalized push notifications, messages and promotions to always keep the bank at the top of customer’s minds. They can also create more targeted marketing campaigns based on the geolocation data to stand out. Even though it might still be best to meet with a human financial advisor, AI-generated financial advice is a great start to improving your current financial situation.
Financial Advice Generation
Another personalization customers expect from banks is financial advice or product recommendations. A report from Salesforce has shown that 66% of customers expect that companies will be aware of their needs and requirements.
Hence, based on information like a customer’s spending habits, financial goals, investment portfolio, risk profile, or budgeting planning, a generative AI can produce wealth management advice, personalized saving plans, or budgeting recommendations. Combining the existing data with data on market trends, your customer can also receive tailored investment or trading advice.
Risk Management and Compliance
The banking and financial sectors have rigid regulations and standards to protect customers and their data, prevent financial crimes, and maintain public confidence. Generative AI is a robust tool for enhancing this aspect of banking.
Fraud Detection and Prevention
Here is how generative AI can help in fraud detection:
- Gen AI can use algorithms to create fake, extra data that mimics fraudulent and non-fraudulent transactions for a bigger dataset. This bigger dataset trains AI for more types of fraud, especially when there isn’t enough existing data on fraudulent activities.
- By analyzing the data and user behaviors, AI can spot transaction anomalies or unusual spending patterns, flagging potential hazards.
- Gen AI constantly enhances its fraud detection capabilities. It constantly learns and adapts to new transaction patterns, user behaviors, or fraud patterns as they are happening in real time.
- Rule-based, traditional systems may gloss over irregular but subtle patterns that may indicate fraudulent activity. Gen AI algorithms analyze a large set of data like login routines, transaction habits or even mouse movements to determine normal user behavior. This personal behavior analysis allows the system to distinguish legitimate and fraudulent activities accurately.
- In addition to using data produced by users, data from sources like industry reports and real-time incidents train GenAI on the latest and evolving fraud schemes.
These innovations are significant, as AI-enhanced scams are worryingly becoming more and more sophisticated. It’s getting harder and harder to tell apart what’s real and what’s not. Scammers can easily pretend to be a CEO or other manager of a business or use their voice and images to fake a video call. The consequences of such malicious attacks aren’t only devastating to individual customers but to the economy as a whole. Cybersecurity Ventures estimated that cybercrime will cost the global economy $9.5 trillion USD in 2024.
Compliance and Regulatory Checks and Reporting
There are multiple regulations banks need to follow, ranging from Know Your Customer (KYC), Anti-Money Laundering (AML), consumer protection laws, and fair lending laws. It goes without saying that the process of checking, analyzing, and drafting compliance reports is time-consuming and tedious. A well-trained generative AI makes sure the basic regulatory and standard requirements are met, thus speeding up customer onboarding and reducing the likelihood of false positives.
Credit Approval and Loan Underwriting
According to a McKinsey report, 80% of credit risk organizations anticipate implementing new AI technology in the next 12 months.
One of the reasons might be that AI can speed up the process of credit approval and loan underwriting significantly by quickly reviewing the documents. It automatically drafts outreach communication should there be missing information from customers and then extracts and compares information. After the credit is approved, Gen AI streamlines the contracting process by drafting legal documents and automatically notifying customers of the completed process.
All in all, AI not only speeds up the process but also ensures the document’s compliance with standards and requirements.
Debt Collection
Debt collections have long been a delicate aspect of customer relations. AI aids this process with advanced analytics. To be specific, the tool can address individual needs effectively while creating tailored payment plans based on the extensive data set. Moreover, gen AI models can detect early willingness to pay based on sentiment analysis, enabling targeted contact strategies and offers.
All in all, based on the information collected, AI automatically delivers the message with the right timing and tone, improving recovery rate and customer relationships. According to a 2023 poll of third-party collectors, 60% were planning to use AI/ML technologies, and 11% were already doing so.
Strategic Decision-Making
Banking is a complex landscape where strategic decisions can make or break an organization’s success. Gen AI provides a powerful tool to navigate customer relationships, safeguard customer data, and synthesize the vast amount of data to facilitate rapid, informed decision-making.
Data-driven Decision Making
According to Seagate’s “Rethink Data” report, 68% of the data businesses have access to is wasted. Banks have access to an ocean of data, yet the inability to effectively analyze and turn data into actions is a massive waste of potential. This might be a result of the lack of resources, expertise, data silos, or outdated systems.
Leveraging generative AI as a part of decision-making gives banks the confidence to make informed decisions while complying with the standards and regulations.
Financial Forecasts
Financial forecasts allow businesses to assess their investment opportunities and minimize risks while optimizing resources. A number of data points go into financial forecasts: historical data, market trends, cash flow, economic indicators, customer metrics, etc. Generative AI enhances financial forecasting by using data and simulating scenarios, enabling teams to strategize and mitigate risks based on future market and economic conditions. Its real-time insights and predictive analytics help businesses identify growth opportunities and improve decision-making efficiency.
Algorithmic Trading
Algorithmic trading, or algo-trading, uses computer programs to execute trades based on predefined rules, such as timing, price, or quantity. It ensures speed, efficiency, and emotion-free decision-making. It aims to optimize trade execution and improve market liquidity while reducing human intervention.
ML and AI can introduce consistency in algorithmic trading. By first collecting and analyzing historical and real-time data (price movements, trading volumes, economic indicators) and identifying trends and relationships, gen AI develops trading strategies. The constant training of AI models will eventually create trading rules, risk management metrics, and buy or sell signals. Based on the rules and strategies, AI will execute trades automatically, minimizing human intervention.
Process Optimization
With its rigid standards, banking professionals need to process an array of documents daily. This ranges from customer-related documents, compliance and regulatory documents, to internal and legal ones Generative AI streamlines this process. For example, the tool extracts relevant information in emails or scanned documents or summarizes long documents. This not only speeds up the overall process but also ensures data accuracy and consistency, compliance with regulations, and enhances customer satisfaction.
Real-Life Use Cases of Generative AI in Banking
We’ve already discussed chatbots as one of the many use cases of generative AI. Let’s zoom in closer to other real-life examples of generative AI in banking. While the two examples above offer a glimpse into gen AI’s potential, they are just a sample of the many ways banks worldwide are leveraging this transformative technology to stay ahead of the competition. From customer service and data security to strategic decision-making, gen AI is driving innovation and redefining the banking landscape.
Mastercard
Mastercard has utilized AI to speed up fraud detection and protect its cardholders. Technology continues to develop rapidly, and so do cyberattacks. They steal card details using advanced spyware, malware, and card skimming. However, it’s hard to detect and catch them, as they only sell partial card details on illegal websites.
With gen AI, banks can quickly scan billions of transactions across cards and merchants and detect sophisticated fraud patterns with the help of predictive technology. AI can also predict full card details to alert banks in time.
Mastercard has reported that it doubled the rate of compromised cards and reduced false positive fraudulent transaction detection by up to 200%. The organization also increased the speed of identifying at-risk merchants by 300%.
JPMorgan Chase
JPMorgan Chase has rolled out an AI assistant called LLM Suite to more than 60,000 employees. The bank wants to empower its employees to focus on more high-value work, as this AI assistant carries several noticeable features, including:
- Access to external large language models like ChatGPT
- Protects the bank’s proprietary data from external exposure
- Teaches employees prompt engineering to maximize AI’s capability
However, JPMorgan did note that they expect a change in the workforce due to automation and that the AI model’s use should be limited to individual customers to avoid misinformation risks.
Challenges of Gen AI in Banking
Gen AI solutions still need 2 to 5 more years of testing. Its benefits and implementation are likely to happen gradually and incrementally.
As promising as this powerful tool is, there are still challenges and limits every financial organization needs to be acutely aware of. They involve three main aspects: Data and system, operational, and human factors.
Data and System Challenges
One of the top concerns relating to generative AI is data privacy and security. Since Gen AI relies heavily on data as its foundation, any flaws, vulnerabilities, or breaches in this foundation can compromise the entire system.
Data Privacy and Security
You’ve probably noticed that Gen AI needs to be fed a large amount of data to perform most of its functions. However, by doing this, banks might unknowingly violate their customers’ data privacy rights. Banking customers’ information is sensitive, and the consequences can be detrimental if a data breach happens. It’s best to implement robust security measures and be transparent about the collected while still complying with security regulations like GDPR and CCPA.
It’s crucial to keep in mind that even though Gen AI is used to streamline and optimize banking tasks, it can also be used for scamming and phishing. Delloite has reported that AI “offers seemingly endless potential to magnify both the nature and the scope of fraud against financial institutions and their customers…” Educate and warn your customers about these possible threats and attacks.
Poor or Incomplete Dataset
Gen AI models rely on accurate and up-to-date datasets to produce precise results. Poor, biased or incomplete data fed to the AI will negatively impact the responses and results known as “AI hallucination”. Simply put, AI models learn to make predictions by identifying patterns in their training data, but the flawed dataset might lead to incorrect assumptions or biased responses.
Operational Challenges
Almost every technology faces operational challenges, and generative AI is no exception. Seamless integration with existing infrastructure, scaling the system to handle increasing demands, or maintenance to ensure reliability are all aspects that, if not done effectively, can slow down adoption and increase operational costs.
Integration with Existing Dataset
Integrating existing legacy banking systems with AI frameworks is a significant challenge. The outdated data structures and protocols might not be compatible with modern AI technology. Another problem is the data silo or propriety forms data is stored in, making it hard to retrieve and analyze data. Dealing with this issue is extremely costly and time-consuming, especially if organizations wish to integrate the models with as little disruption to the current operations as possible.
Scalability and Maintenance
Similar to other modern technologies, generative AI models need constant maintenance and updates to adapt to changing business and market needs. Scaling AI solutions can be resource-intensive, so make sure you have the right resources and support in place.
Human and Perception Challenges
Gen AI may be the technology of the future, but it cannot reach its full potential without human expertise and oversight. Yet, humans might be the very challenge that hinders gen AI from operating effectively. Whether it’s rules and regulations, a shortage in specialized skills or a lack of trust, these human factors can limit AI’s full potential to deliver on its promise of increased efficiency.
Regulatory Compliance
Banking is a sector with strict regulations and laws. Even though generative AI is still mostly unregulated, its adoption needs to meet all the basic standards and regulations of the industry, including financial laws, data security and protection laws, etc.
Talent Shortages
Building, maintaining and operating a generative AI model requires specialized skills. However, the market is witnessing significant skill gaps. A startling 60% of public sector IT professionals cited a lack of AI skills as their biggest obstacle to deploying AI in a recent Salesforce survey. This means banks might need to invest in training and hiring to bridge this gap or resort to outsourcing to find these specialized skills.
Public Perception and Trust
Since generative AI is a new technology, banks need to ensure public trust. Financial institutions need to help the public recognize AI’s positive transformational impact on the industry. You don’t want to create distrust and eventually lose customers because they, for example, don’t think the technology is safe enough. Again, the key here is to be transparent about how customer data is used and let your customers know the benefits of having generative AI onboard in banking services.
An Overview of Gen AI Operating-model Archetypes
Choosing a suitable generative AI model plays a large part in determining its success in the enterprise. Each bank may take different approaches to this topic, but the model should be able to scale and align with the organization’s culture and structure. A suitable model should also be able to align with the enterprise’s strategic objectives, define common standards for technology architecture, practices, and risk frameworks, and put use cases into production.
There are four main generative AI archetypes, ranging from highly centralized to highly decentralized.
Highly Centralized
A highly centralized gen AI operation archetype consists of a team that holds the main responsibility over gen AI solutions. The team is independent from the rest of the enterprise, which creates space for skills and quick building for the team. However, the team is also siloed from the decision-making process and other business functions.
Centrally Led, Business Unit Executed
With more collaboration from the business units and the AI team, this archetype reduces the resistance to enterprise-wide adoption of the AI model. However, the AI team may need sign-offs and approval from the business units, possibly slowing down the overall process.
Business Unit-led, Centrally Supported
Even though this archetype makes it simple to gain business support from departments (as the techniques are implemented from the bottom up), the differing levels of gen AI may hinder the process itself.
Highly Decentralized
In a highly decentralized model, business functions readily support the technology. Gaining relevant insights for specialized resources doesn’t take much time. Businesses that handle their gen AI technology alone, however, risk missing out on best practices and expertise that may be obtained through a more centralized approach. Breakthroughs are consequently more difficult as it’s harder for teams to dig deep.
Transformative Future of Generative AI in Banking
While AI has great potential to revolutionize banking, it should be viewed as a powerful assistant, not the ultimate decision-maker. As gen AI continues to evolve, banks will increasingly harness its power to streamline operations, improve customer experiences, and drive innovation.
Now is the perfect time to not only test the waters but also start exploring how a gen AI model can benefit you. Don’t wait for others to reap the benefits of gen AI in banking - seize this opportunity to take your organization to new heights of success and customer satisfaction. Start chatting with experts in the field and see how your organization can start embracing AI technology!